Machine Learning is becoming democratized.
Having worked on AWS tools, I know how planning a simple click can cost people a lot of money when it comes to using developer tools.
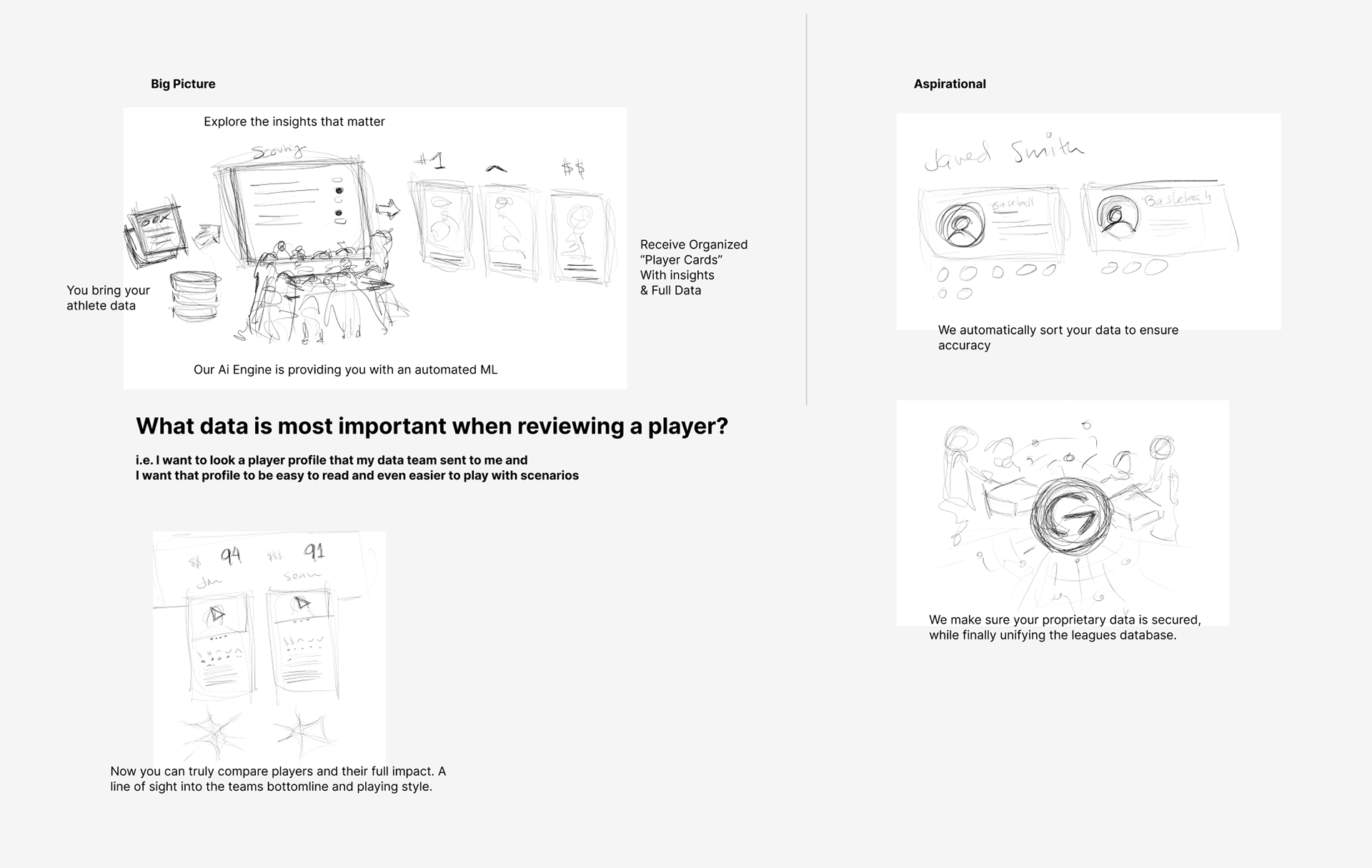
This allowed me to focus on making a single project very simple to run. If someone can train a data set right away, what would that look like.
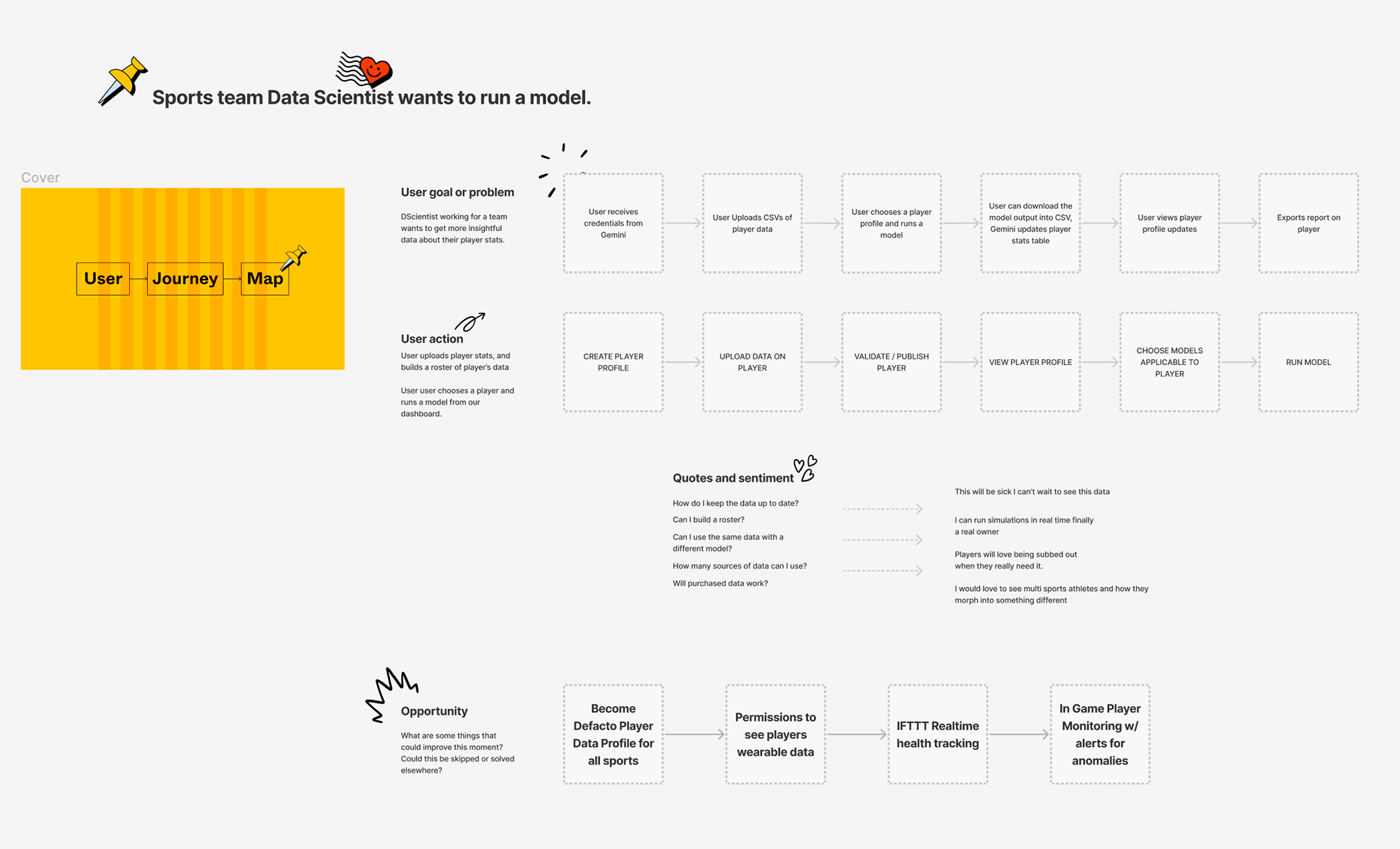
Let's outline the requirements and then see if the flow supports it.
I also like to sketch what needs could be glaring from the user.
I also like to sketch what needs could be glaring from the user.
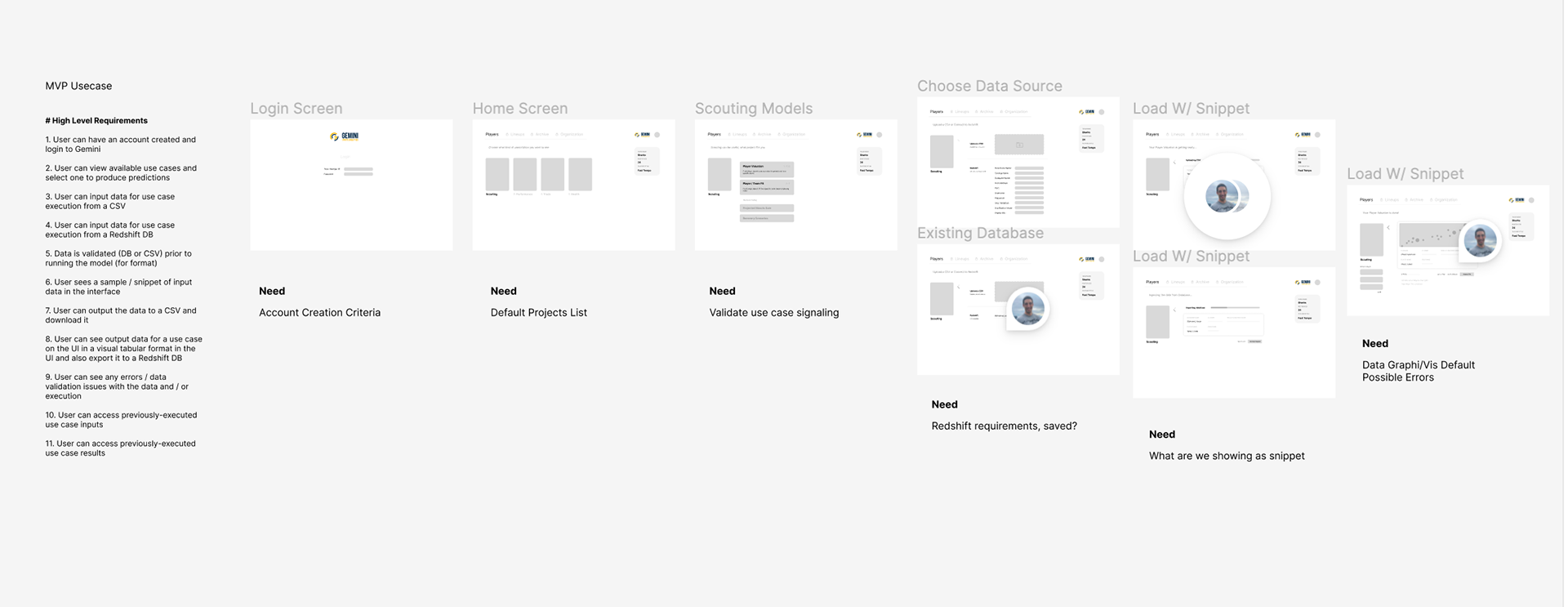
It's also fun working backwards. I like to make a few home pages to see if the message can be simplified.
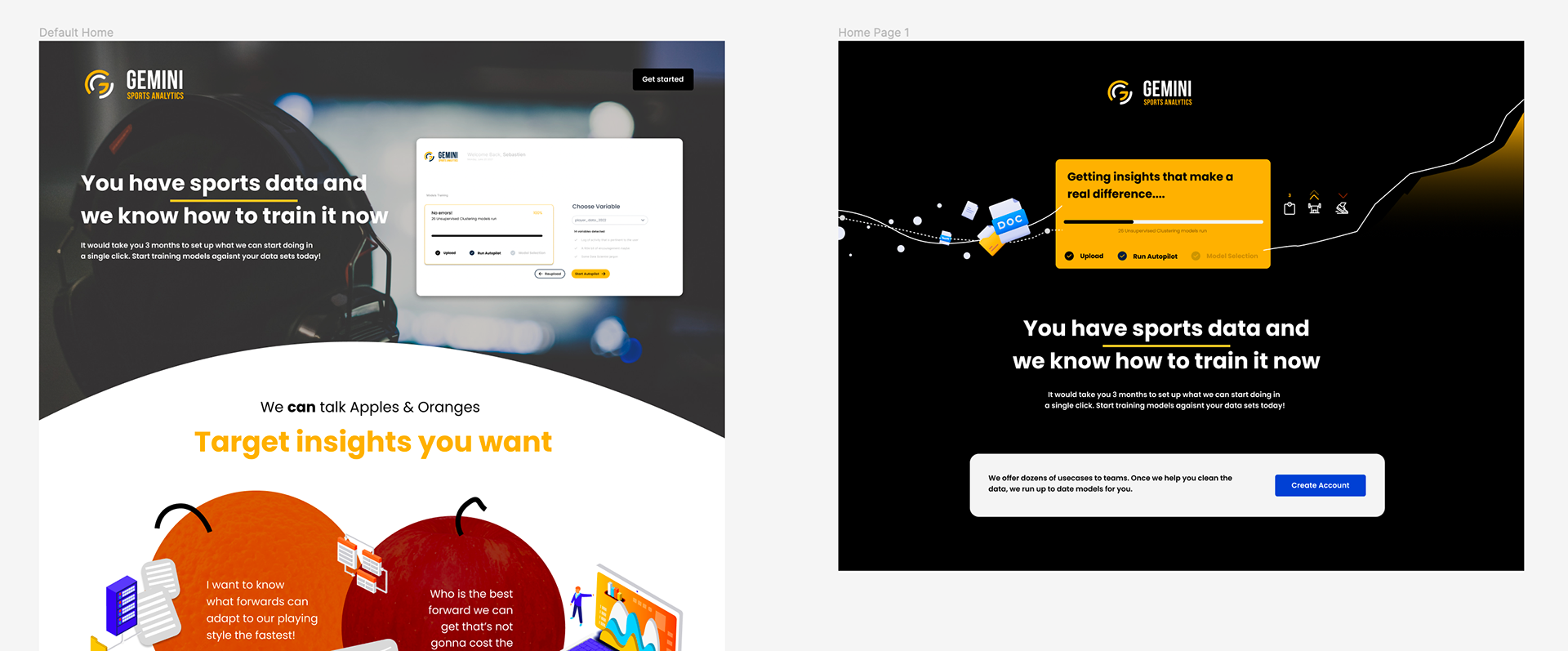
Now let's focus... Worked with team to outline Epics that Design can align with. Priority allows us to see what features will be available.
Creating an ML project...
Creating an ML project...
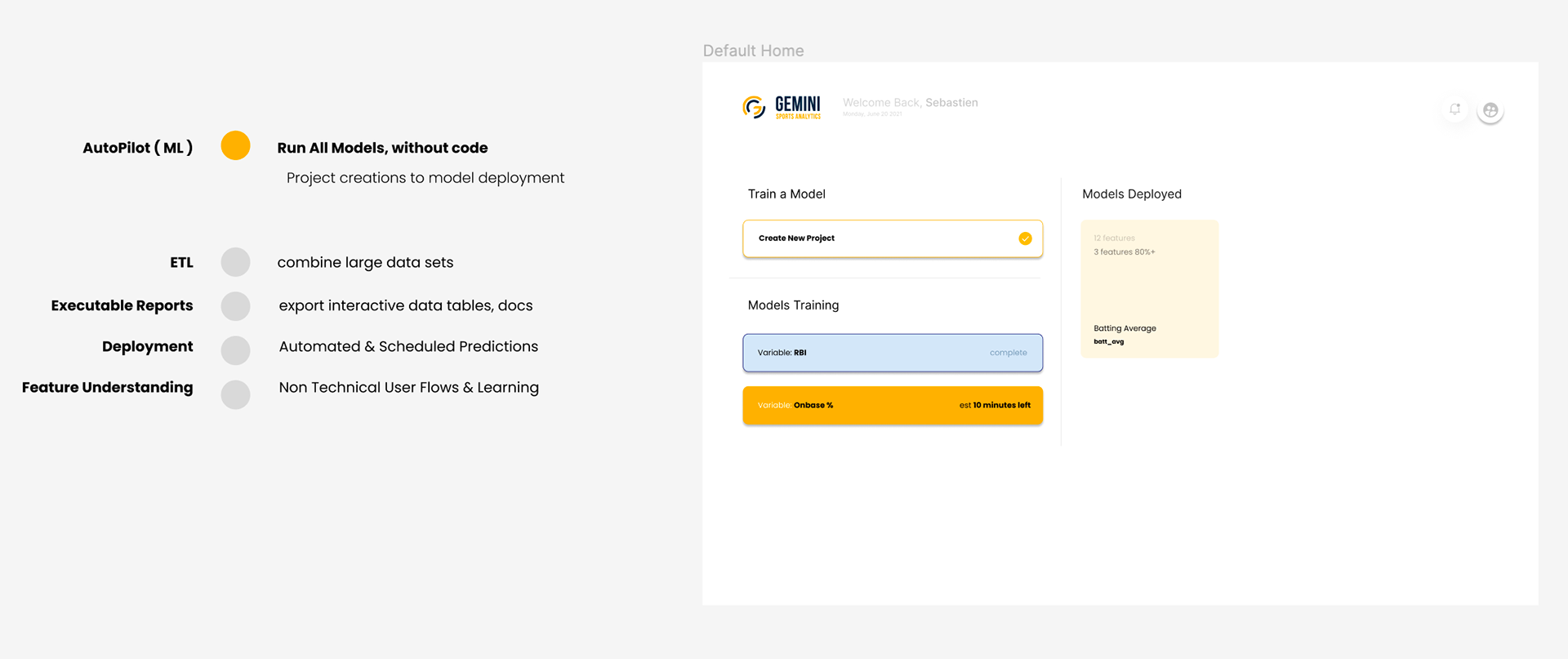
I was brought in to simplify a very complicated Data Scientist process and give sports teams and even GMs a look at the data they have, but trained.